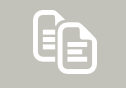
Stacked Autoencoders for the P300 Component Detection
Using a combination of unsupervised pre-training and subsequent fine-tuning, deep neural networks have become one of the most reliable classification methods. The aim of the experiments subsequently presented was to verify if deep learning-based models can also perform well for single trial P300 classification with possible application to P300-based brain-computer interfaces. The P300 data used were recorded in the EEG/ERP laboratory at the Department of Computer Science and Engineering, University of West Bohemia, and are publicly available. Stacked autoencoders were implemented and compared with some of the currently most reliable state-of-the-art methods, such as LDA and multi-layer perceptron. The parameters of stacked autoencoders were optimized empirically. Subsequently, fine-tuning using backpropagation was performed. The architecture of the neural network was 209-130-100-50-20-2. The classifiers were trained on a dataset merged from four subjects and subsequently tested on different 11 subjects without further training. The trained SAE achieved 69.2% accuracy that was higher (p < 0.01) than the accuracy of MLP (64.9%) and LDA (65.9%). The recall of 58.8% was slightly higher when compared with MLP (56.2%) and LDA (58.4%).